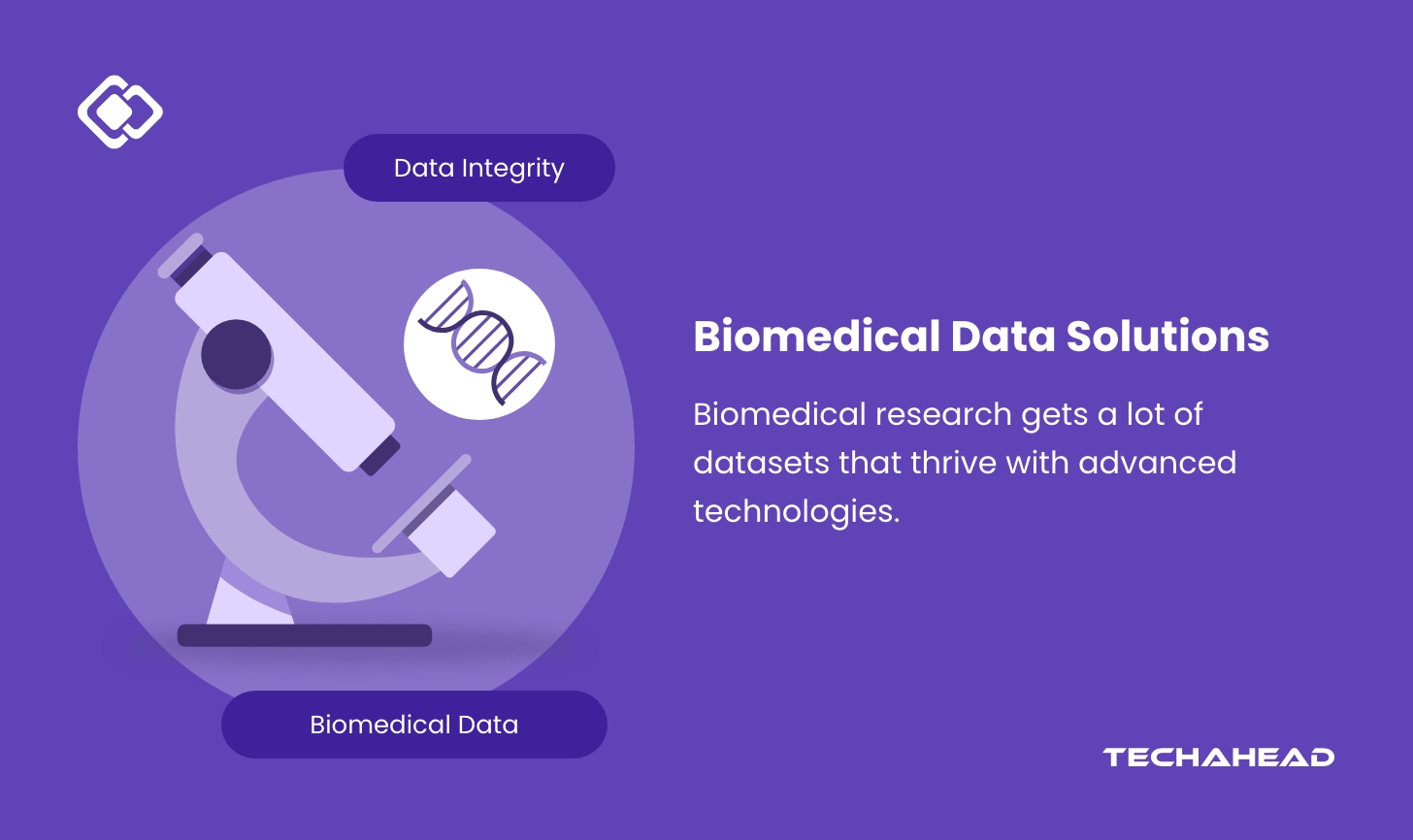
Modern biomedical research generates vast volumes of complex data. High-throughput technologies produce terabytes of information daily, spanning genomics, proteomics, and multi-omics datasets. Seamlessly connecting the discovery and development phases is essential for innovation, ensuring that promising findings transition smoothly into real-world applications.
The global clinical data management system market exemplifies the growing importance of efficient data solutions. Valued at USD 2.79 billion in 2023, it is projected to reach USD 8.13 billion by 2033, expanding at a CAGR of 11.28% from 2024 to 2033. This surge highlights the increasing demand for reliable management of clinical trial data, driven by the need for accuracy and streamlined processes in biomedical research.
Scalable data solutions offer a path to efficiency, accuracy, and cross-functional collaboration, addressing the challenges of managing biomedical data. By enabling seamless integration and fostering innovation, these solutions pave the way for groundbreaking advancements in biopharma and precision medicine. Organizations adopting these technologies can unlock unparalleled potential, ensuring they remain at the forefront of discovery and development.
Volume and Complexity of Biomedical Data
Modern biomedical research thrives at the crossroads of genomics, proteomics, and clinical datasets. Advanced high-throughput technologies generate vast amounts of data, often in terabytes per dataset. For instance, a single whole-genome sequencing experiment produces over 200 gigabytes of raw data. This vast scale is matched by the diversity of data types, including single-cell RNA sequencing, multi-omics studies, and electronic health records (EHRs). Each dataset brings unique challenges, requiring tailored approaches for effective integration and analysis.
The complexity of biomedical data adds another layer of difficulty. These datasets are not only multi-dimensional but also interdependent, demanding advanced tools for annotation, metadata tagging, and harmonization. Standardization across repositories remains a major challenge due to varied file formats and data schemas. This lack of uniformity complicates efforts to merge and analyze data seamlessly. Addressing these challenges by providing innovative solutions for biomedical research and biopharmaceutical organizations ensures that data is effectively managed and utilized.
Integration Challenges Across Research Phases of Biomedical
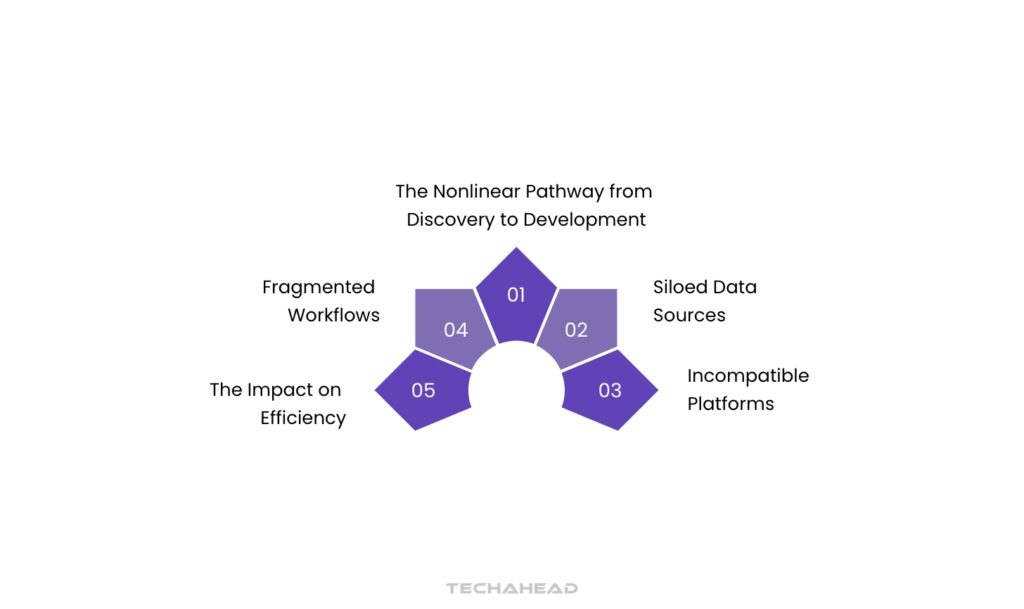
The journey from discovery to development is rarely straightforward. Research organizations face significant hurdles, including siloed data sources, incompatible platforms, and fragmented workflows. These challenges create major roadblocks in maintaining seamless data continuity across various research phases.
The Nonlinear Pathway from Discovery to Development
The transition from discovery to development is rarely straightforward. Each phase generates unique challenges, disrupting the flow of data and processes.
Siloed Data Sources
Data from various research phases is often stored in isolated systems. This lack of interconnectedness prevents teams from accessing or sharing critical insights. These silos hinder collaboration and delay progress.
Incompatible Platforms
Different departments rely on diverse tools and technologies that rarely integrate seamlessly. The lack of standardization requires significant effort to convert and harmonize data across platforms, causing inefficiencies.
Fragmented Workflows
Research workflows are frequently disjointed, especially during transitions between discovery, preclinical, and clinical phases. These handoffs are prone to miscommunication, errors, and delays, impacting timelines and operational costs.
The Impact on Efficiency
Disrupted data continuity and fragmented processes lead to wasted time and resources. Without streamlined integration, the entire research-to-development pathway becomes slower and less effective.
Overcoming these challenges requires a strategic approach. Standardized data formats, unified platforms, and collaborative workflows are essential for maintaining seamless transitions and boosting efficiency.
Challenges in Biomedical Data Management
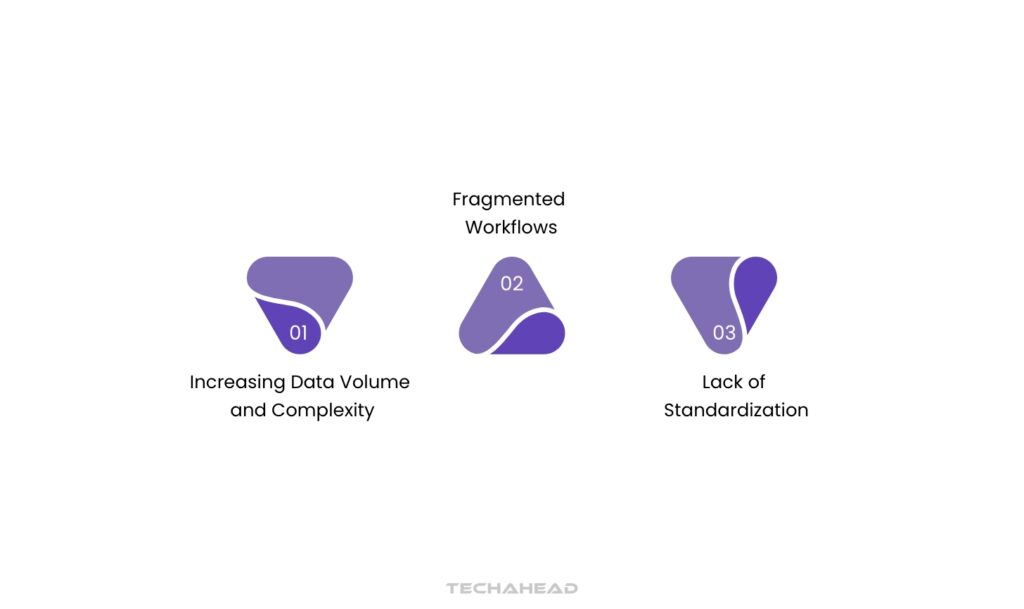
Increasing Data Volume and Complexity
Biomedical research operates at the intersection of diverse disciplines, producing enormous datasets. For example, a single whole-genome sequencing experiment generates over 200 gigabytes of raw data. This complexity intensifies with the integration of multi-omics data, such as proteomics and transcriptomics, creating challenges for storage, processing, and analysis.
Fragmented Workflows
Data silos remain a major barrier in biomedical research, disrupting collaboration between teams. The lack of a cohesive approach slows the transition between discovery and development. As a result, valuable insights often remain underutilized, with inefficiencies emerging from disconnected workflows and limited data continuity.
Lack of Standardization
The absence of consistent file formats and data schemas across repositories creates significant obstacles to integration. These inconsistencies lead to duplicated efforts, misinterpretation of results, and difficulties in scaling operations. Standardization is critical for harmonizing data pipelines and ensuring reliable outcomes.
Role of Scalable Solutions in Biomedical Research
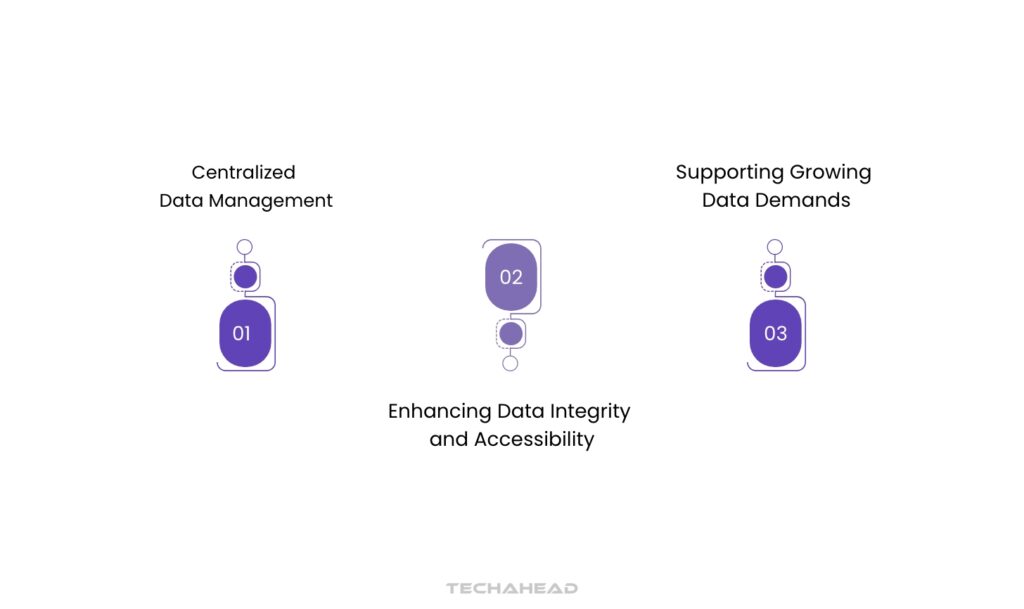
Centralized Data Management
Unified storage and automated processing are pivotal in managing biomedical data. Scalable cloud-native platforms simplify workflows, enabling teams to focus on innovation rather than infrastructure. For instance, centralizing genomics and proteomics data on a single platform reduces redundancies and streamlines access.
Enhancing Data Integrity and Accessibility
Automation minimizes manual intervention, reducing errors while ensuring data consistency. Real-time availability of data allows interdisciplinary teams to collaborate seamlessly, fostering innovation and timely decision-making. Centralized platforms also enhance compliance with data governance standards.
Supporting Growing Data Demands
Scalability is essential for handling ever-increasing data volumes. Platforms designed for high-throughput environments ensure robust performance, whether processing terabytes of sequencing data or managing longitudinal clinical datasets. This adaptability is vital for keeping pace with technological advancements and research demands.
Bridging Discovery and Development with Scalable Biomedical Tools
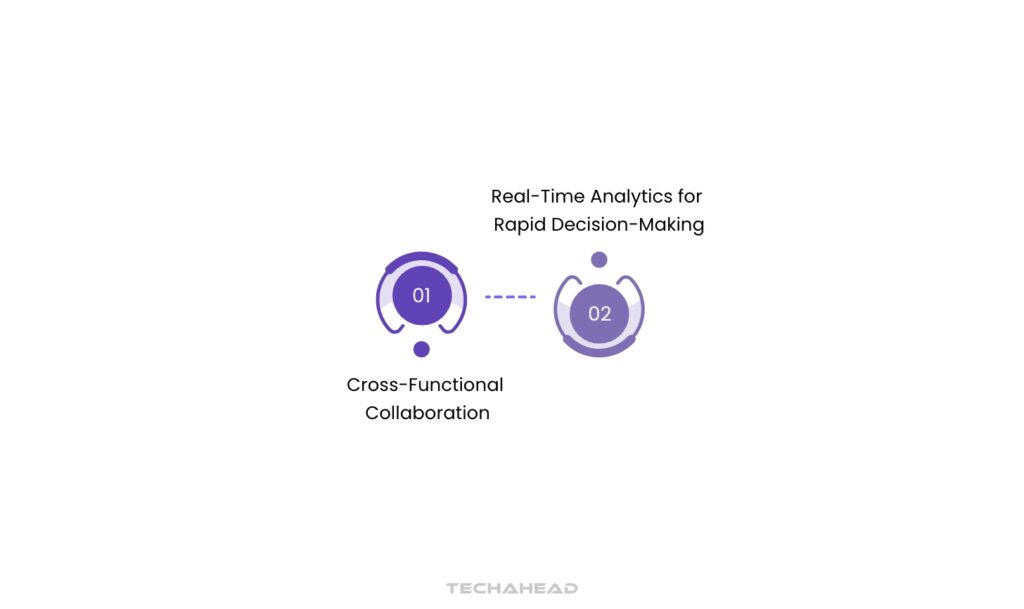
Cross-Functional Collaboration
Biomedical innovation thrives on diverse expertise, from biologists to data scientists. Integrating these disciplines requires clear communication and unified workflows. Scalable solutions foster collaboration by providing centralized platforms where all stakeholders can contribute and access data efficiently.
Real-Time Analytics for Rapid Decision-Making
Machine learning accelerates the analysis of complex datasets, enabling predictive modeling and hypothesis testing. Tools offering interactive visualizations and real-time monitoring empower researchers to make data-driven decisions, enhancing the transition from discovery to development.
Benefits of Scalable Biomedical Data Solutions
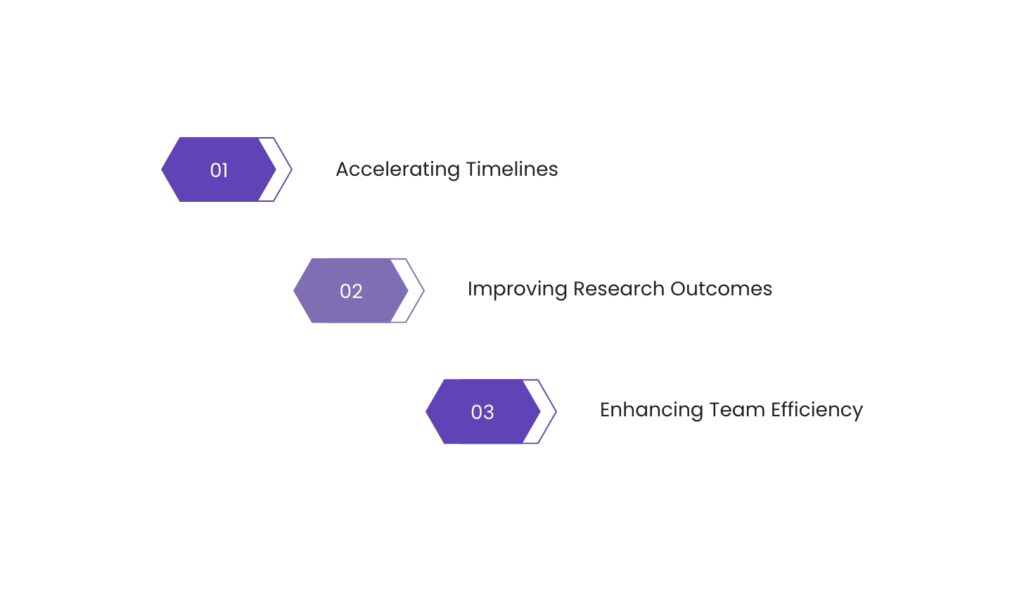
Accelerating Timelines
By eliminating fragmented workflows and manual processing, scalable solutions reduce delays. Streamlined operations ensure discoveries are rapidly translated into development milestones.
Improving Research Outcomes
Scalable platforms maintain data integrity and provide real-time insights, enabling informed decisions. This precision enhances the reliability of research outcomes, driving innovation.
Enhancing Team Efficiency
Collaborative workflows and optimized resource utilization allow teams to focus on strategic objectives. Scalable solutions free researchers from technical bottlenecks, maximizing productivity.
Conclusion
The adoption of scalable solutions delivers exceptional returns on investment (ROI) for biopharma and research organizations. Since 2015, we have pioneered innovative approaches to managing data infrastructure, consistently achieving measurable ROI and tangible impacts. Our collaborations with top biopharma companies show that seamless data integration significantly reduces analysis time while improving research accuracy and outcomes.
What makes us unique in this domain is our deep expertise in both technical challenges and biological intricacies. We understand how robust data architecture not only streamlines operations but also empowers scientists to focus on innovative research. A well-structured system reduces inefficiencies, enabling breakthroughs.
Our impact extends far beyond operational improvements. Research teams have reported faster hypothesis development, enhanced reproducibility of findings, and accelerated time-to-insight. These advancements directly translate into shorter research timelines and optimized research budgets. Streamlined processes mean more resources for discovery.
By eliminating bottlenecks and fostering collaboration, TechAheads healthcare development solutions have transformed research landscapes. These changes not only drive efficiency but also open doors to groundbreaking discoveries, enabling biopharma organizations to push the boundaries of innovation.
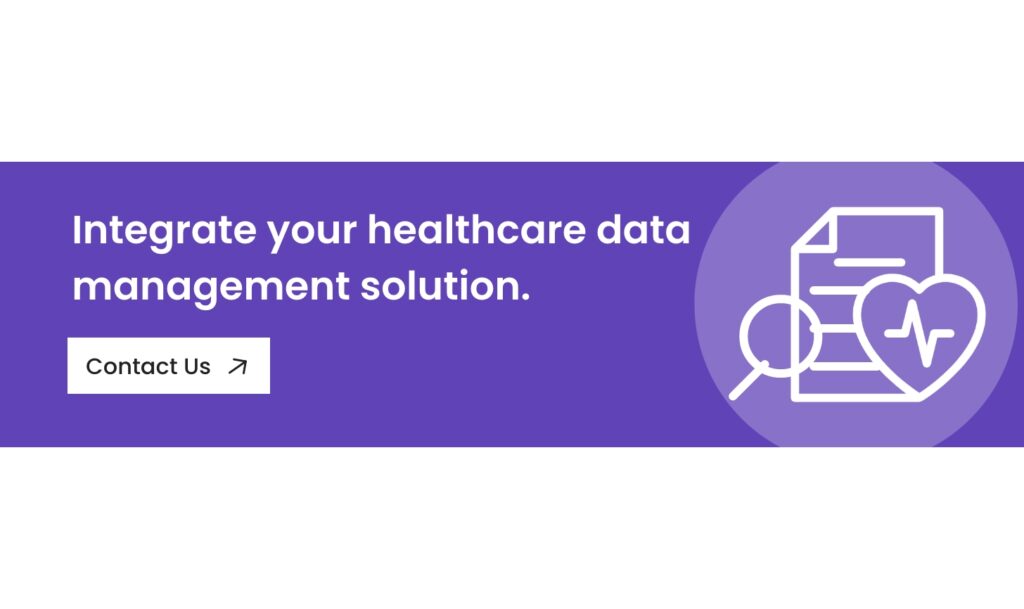
FAQs
Biomedical data encompasses various forms from diverse sources. Examples include images from CAT and MRI scans, EEG signals, laboratory data such as blood and specimen analyses, and patient clinical records.
Healthcare data analysis spans multiple approaches, including descriptive, diagnostic, predictive, and prescriptive analytics. Each type plays a role in understanding, predicting, and improving patient outcomes.
AI applications in biomedical research are vast. Examples include early diagnosis of Alzheimer’s disease through brain network analysis, blood glucose prediction using wearable sensors, enhanced colorectal cancer screening via advanced image analysis, and smart clothing designed to alleviate lower back pain.
AI revolutionizes biomedical engineering by transforming healthcare delivery. It aids in diagnosing diseases, identifying optimal treatments, and predicting patient outcomes, significantly enhancing medical practices.
Biomedical engineering continues to evolve with groundbreaking advancements. Exciting trends include telesurgery for remote operations, tissue engineering for research innovations, and medical virtual reality tools for creating precise patient models and images