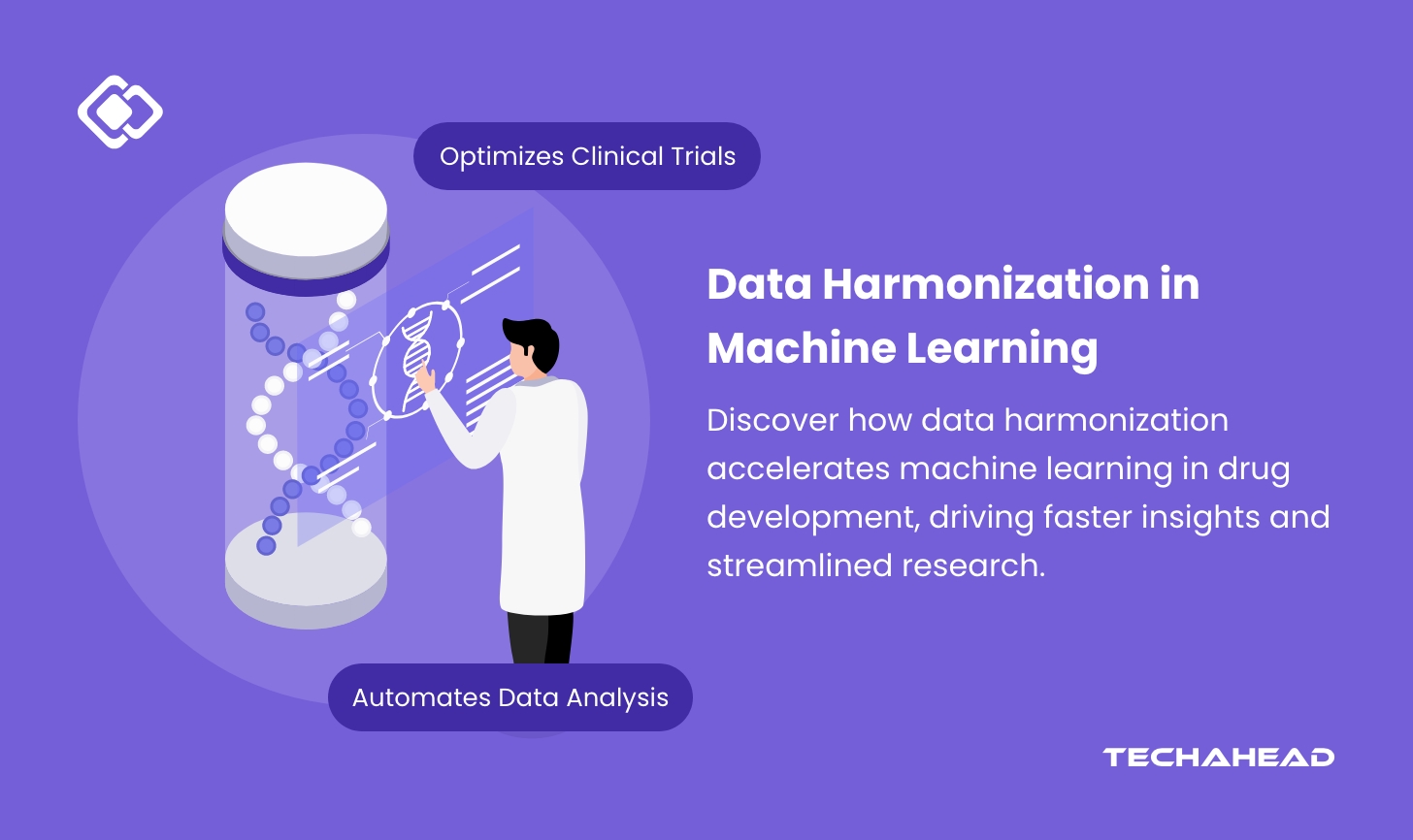
In the expansion of drug development, inconsistent data formats & fragmented datasets can significantly hamper the effectiveness of machine learning (ML) models. A staggering 80% of healthcare data remains unstructured, making it challenging to harness its full potential.
The COVID-19 pandemic highlighted the urgent need for harmonized clinical & genomic data to speed up drug discovery.
For instance, AstraZeneca utilized harmonized datasets to enhance its AI-driven models, identifying favourable new drugs for COVID-19. Similarly, Pfizer’s machine learning models relied on data harmonization to improve clinical trial outcomes.
Without such harmonized data, breakthroughs in drug development would face substantial obstacles, stifling innovation & adversely affecting patient outcomes.
This blog ferrets into the pivotal role of data harmonization in enhancing the efficiency & accuracy of ML models in drug development, showcasing how it can transform the landscape of pharmaceutical research & improve healthcare delivery.
Key Takeaways:
- Standardized data enables accurate and reliable machine learning.
- Consistent formats allow comprehensive analysis, revealing deeper insights.
- Predicting drug interactions improves safety and informs treatment.
What is data harmonization and why is it crucial in drug development?
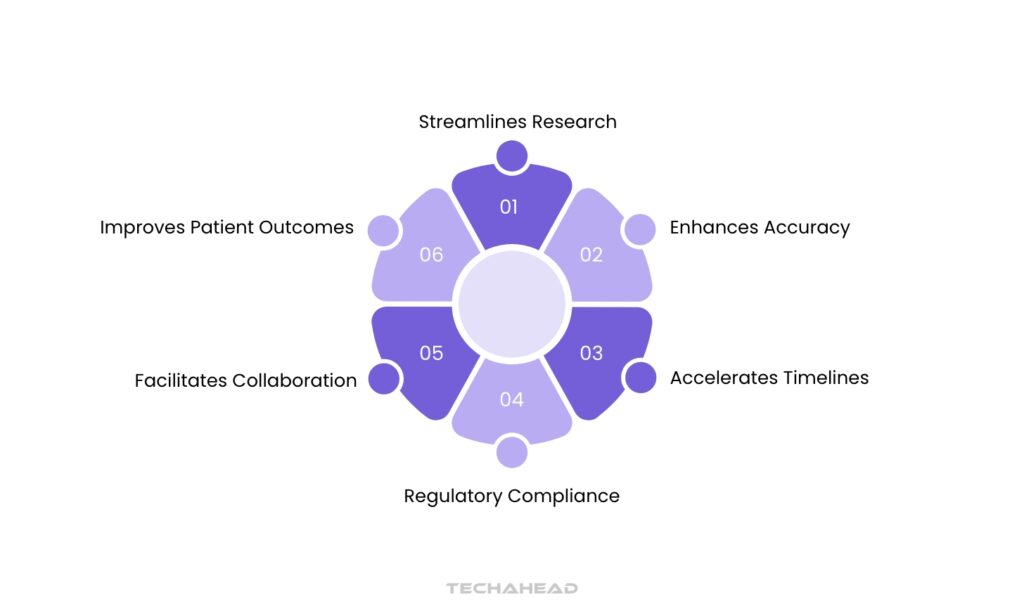
Data harmonization is the process of standardizing data from various sources to ensure compatibility & suitability for analysis. This is particularly important in drug development, as it allows researchers to explore & validate new ideas & technologies effectively.
In the initial phases of research and development, a lot of data proceeds from various experiments, some done with the company & some taken from public databases.
To make sense of this diverse data, harmonization involves filling in missing information & creating uniform data structures, resulting in high-quality datasets that facilitate deeper analyses. This process is crucial for advancing biological research into disease mechanisms, identifying potential drug targets, and discovering new treatment options.
Even though healthcare organizations have tons of data, both organized & unorganized, they often keep it locked away in separate systems, making it hard to get a complete picture of a patient’s health.
This fragmentation hinders research efforts, especially for studies on rare diseases that require larger datasets for robust analysis. By harmonizing data, researchers can address important questions and support evidence-based policy changes in healthcare.
Over the years, the field has evolved from simple digitization to more sophisticated methods of data acquisition and standardization using Common Data Models (CDMs). Effective data harmonization enhances collaboration among researchers and promotes timely communication across stakeholders, ultimately driving innovation in drug development.
The role of machine learning in modern drug development
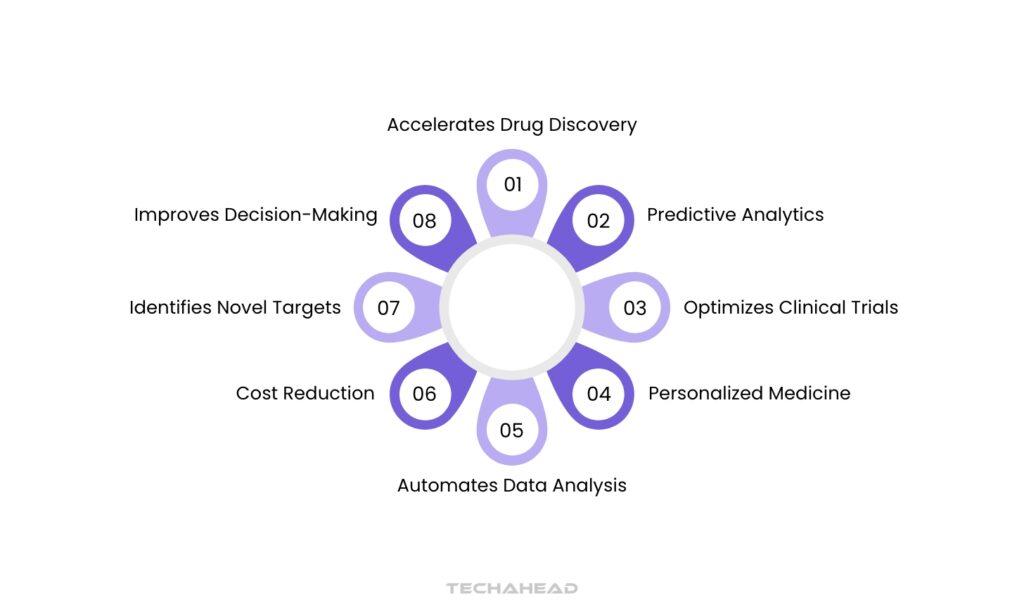
Machine learning offers a much more efficient approach to drug discovery. Instead of traditional, time-consuming methods, powerful computer algorithms can analyze huge amounts of data about chemical structures, their properties, and how they behave biologically.
This analysis allows the algorithms to predict how different chemicals will interact with specific parts of the body that are targeted by drugs.
This predictive ability is a huge advantage. It lets researchers focus on the most promising potential drugs right from the start, saving a lot of time and money that would otherwise be wasted on less promising options. By concentrating on the compounds most likely to work, the path to clinical trials and new treatments becomes much faster.
Beyond speeding up the process, machine learning also helps make drugs safer. These algorithms can analyze data to predict how different drugs might interact with each other in the body.
This helps identify combinations of medications that could cause dangerous side effects. This predictive power helps prevent harmful reactions and empowers doctors to make better, more informed decisions about patient treatment, ultimately leading to improved care.
Challenges in drug development data: Why Harmonization Matters
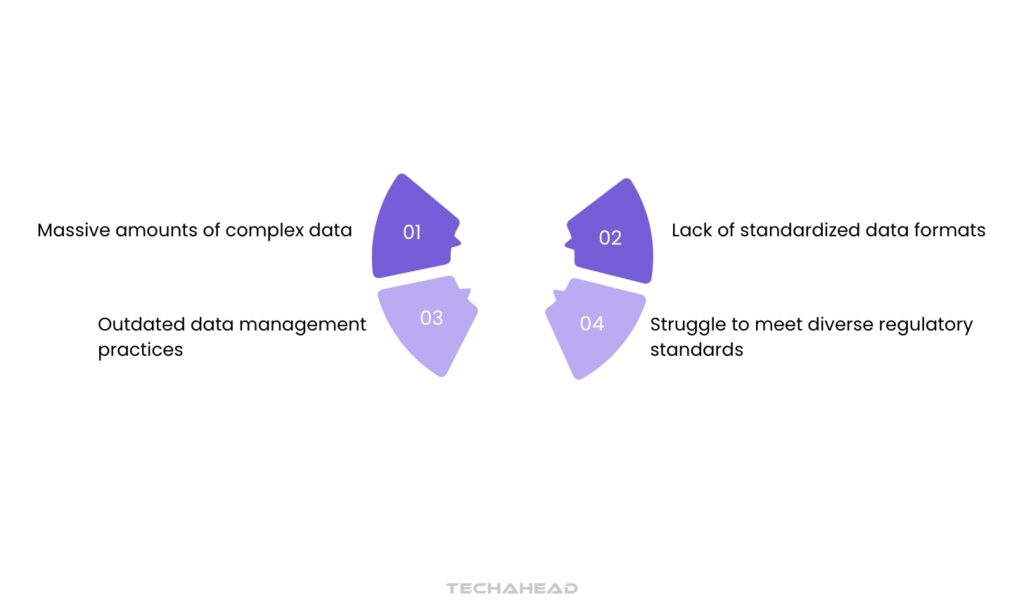
Harmonized data standards enable better collaboration between stakeholders, facilitate more efficient regulatory submissions, and support advanced analytics capabilities. When data is properly standardized, organizations can more easily apply artificial intelligence and machine learning techniques to identify patterns, predict outcomes, and accelerate the drug development process while maintaining high quality standards and regulatory compliance. Below we’ve mentioned the challenges:
Massive amounts of complex data
Drug development generates massive amounts of complex data across multiple stages, from preclinical studies to clinical trials. This data comes from diverse sources including laboratory experiments, patient records, adverse event reports, and real-world evidence, creating significant challenges in maintaining consistency & compatibility across different formats and systems.
Lack of standardized data formats
The lack of standardized data formats & structures across pharmaceutical companies, research institutions, and regulatory bodies leads to inefficient data sharing & analysis. When datasets use different terminologies, measurement units, or recording methods, it becomes extremely difficult to aggregate and compare results effectively, potentially slowing down the drug development process.
Outdated data management practices
Legacy systems and outdated data management practices within organizations often create data silos, making it challenging to integrate historical data with newer information. This fragmentation can result in missed insights and duplicated efforts, ultimately increasing the time and cost of bringing new drugs to market.
Struggle to meet diverse regulatory standards
Regulatory compliance requirements vary across different regions and jurisdictions, necessitating careful attention to data documentation and traceability. Without proper harmonization, companies struggle to meet diverse regulatory standards while maintaining data integrity and consistency throughout the drug development lifecycle.
How Data Harmonization Enhances Machine Learning Models in Drug Development
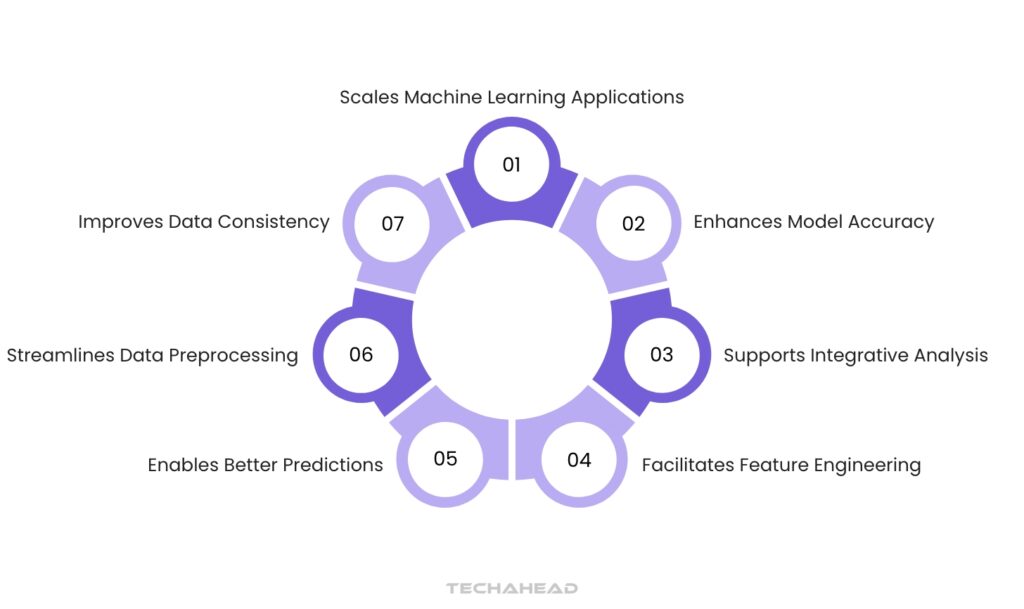
Data harmonization plays a vital role in enhancing machine learning (ML) models within drug development by ensuring that diverse datasets are standardized and integrated effectively.
In the drug discovery process, researchers often encounter fragmented data from various sources, which can lead to inconsistencies and inaccuracies. By harmonizing this data, researchers can create a unified dataset that improves the reliability & performance of ML algorithms.
The process of data harmonization involves cleaning, standardizing formats, and normalizing data to minimize biases. This ensures that ML models are trained on high-quality, consistent data, leading to more accurate predictions regarding drug efficacy and patient responses.
For instance, during the COVID-19 pandemic, top R&D companies utilized harmonized datasets to accelerate their drug development efforts, demonstrating the critical impact of data harmonization on timely healthcare solutions.
Moreover, harmonized data facilitates better collaboration among research teams by providing a common framework for analysis. This collaborative approach is essential for addressing complex biomedical questions that require comprehensive datasets.
Ultimately, effective data harmonization not only enhances the accuracy of ML models but also accelerates the overall drug development process, paving the way for innovative therapies and improved patient outcomes. As the field continues to evolve, prioritizing data harmonization will be crucial for leveraging the full potential of machine learning in advancing healthcare solutions.
Benefits of Data Harmonization for Drug Discovery & Clinical Trials
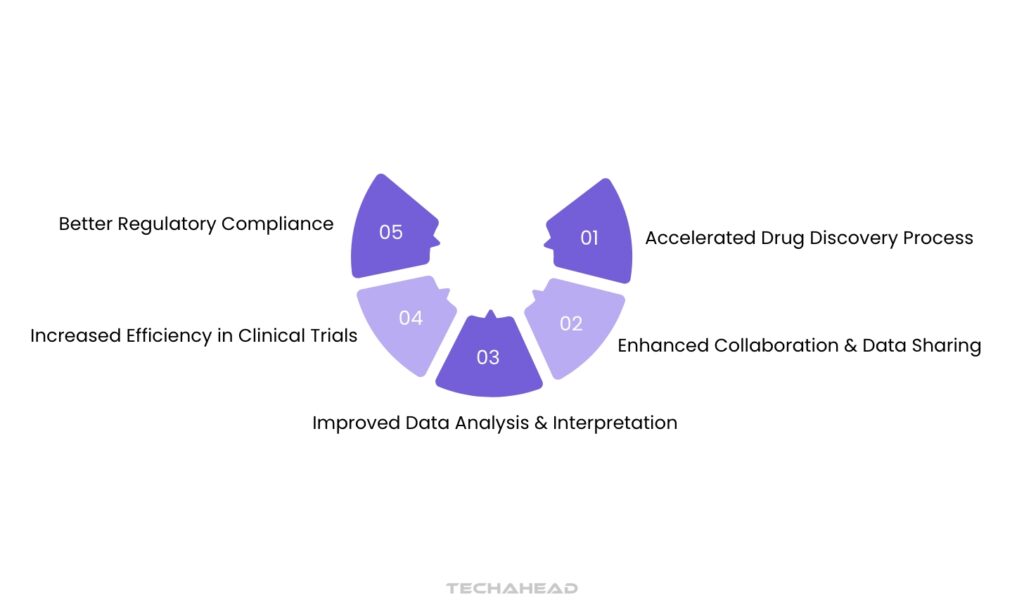
Data harmonization, the process of standardizing data from various sources into a consistent format, offers significant advantages for drug discovery and clinical trials. By creating a unified and accessible dataset, researchers can unlock valuable insights and accelerate the development of new treatments. Here’s how:
Improved Data Analysis & Interpretation
Harmonized data allows for more comprehensive and accurate analysis. Researchers can combine data from different studies, labs, or databases, overcoming inconsistencies and biases that might arise from varying data collection methods. This leads to more reliable conclusions and a deeper understanding of disease mechanisms and drug effects.
Enhanced Collaboration & Data Sharing
Standardized data facilitates collaboration between researchers, institutions, and even across international borders. Sharing harmonized datasets becomes much easier, fostering open science and accelerating the pace of discovery. This is particularly crucial in areas like rare diseases where data might be scattered across various small studies.
Accelerated Drug Discovery Process
By enabling more efficient data analysis, harmonization helps identify promising drug candidates more quickly. Researchers can use harmonized data to build predictive models, identify biomarkers, and understand drug-target interactions, streamlining the drug discovery pipeline.
Increased Efficiency in Clinical Trials
Harmonized data can improve the design and execution of clinical trials. It allows for better patient stratification, more accurate data monitoring, and more efficient data analysis, ultimately reducing the time and cost associated with bringing new drugs to market.
Better Regulatory Compliance
Standardized data formats make it easier to comply with regulatory requirements for data submission and reporting, simplifying the approval process for new drugs and therapies.
Real-World Examples: Data Harmonization in Drug Development Success Stories
Real-world success stories, such as the rapid development of COVID-19 therapies, underscore the critical role of data harmonization in advancing healthcare. Harmonized data provides the necessary infrastructure to support essential tasks like patient stratification, biomarker discovery, and target identification.
By integrating diverse datasets into a cohesive format, researchers can leverage this information to drive innovation and improve patient outcomes.
TechAhead is at the forefront of this movement, offering tailored solutions for data harmonization that utilize advanced technologies. Our platform enables researchers worldwide to overcome the challenges posed by fragmented data sources.
By ensuring that datasets are standardized and accessible, TechAhead empowers scientists to extract meaningful insights from their research.
The importance of harmonized data cannot be overstated; it enhances the efficiency of machine learning models and accelerates the drug development process. As healthcare continues to evolve, the ability to harmonize data will play a pivotal role in shaping future therapies and improving healthcare delivery on a global scale.
With TechAhead’s expertise, researchers can confidently navigate the complexities of data harmonization and focus on what truly matters: advancing healthcare innovations.
The Future of Machine Learning in Drug Development with Data Harmonization
The future of drug development is poised for a significant transformation through the combined power of machine learning and data harmonization. As machine learning algorithms become increasingly sophisticated, their ability to analyze complex biological data and identify promising drug candidates will be greatly enhanced by the availability of harmonized datasets.
Data harmonization, by standardizing data from diverse sources, creates a unified and consistent foundation for machine learning models. This eliminates inconsistencies and biases that can hinder accurate analysis, leading to more reliable predictions of drug efficacy and safety.
This synergy will accelerate the entire drug development pipeline, from target identification and lead optimization to clinical trials and regulatory approval. Ultimately, this powerful combination promises to deliver new treatments to patients faster and more efficiently.
Data Privacy & Security in Harmonized Drug Development Data
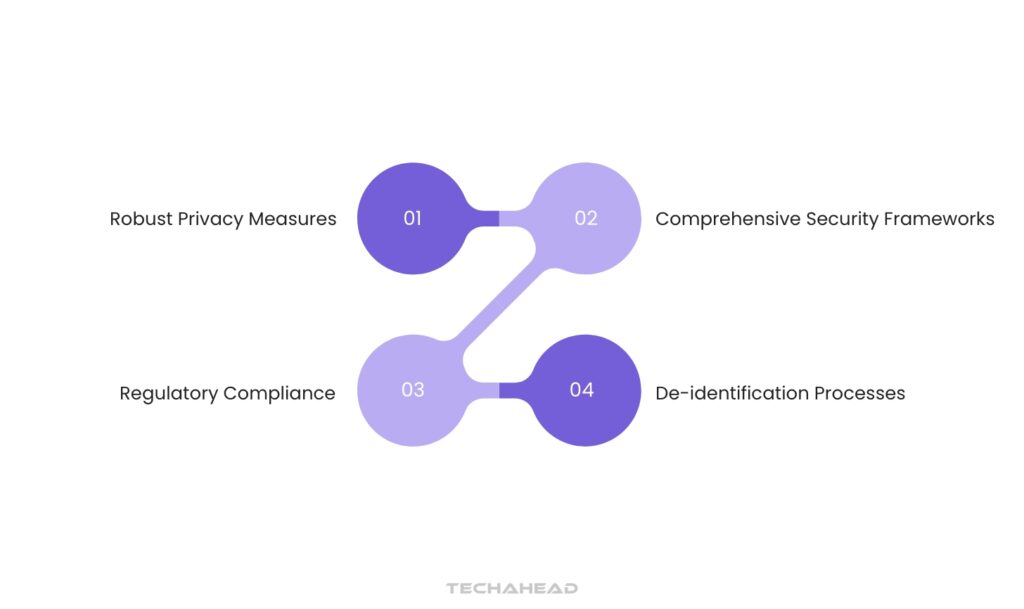
Drug development data harmonization presents unique challenges in maintaining robust privacy and security measures while enabling efficient data sharing and analysis. Protecting sensitive information, including patient data, intellectual property, and proprietary research findings, requires sophisticated security protocols that don’t compromise data accessibility and utility.
Organizations must implement comprehensive security frameworks that account for data encryption, access controls, and audit trails while ensuring compliance with international privacy regulations like GDPR and HIPAA.
This involves establishing secure data sharing mechanisms that maintain data integrity while protecting confidential information across different stakeholders and systems. The challenge extends to managing de-identification processes that preserve data utility while preventing re-identification risks.
As drug development becomes increasingly collaborative, organizations must balance the need for data transparency with privacy protection, implementing technical and organizational measures that safeguard sensitive information throughout the data lifecycle. This includes securing data during transfer, storage, and analysis while maintaining its value for research and development purposes.
How Data Harmonization Impacts Regulatory Approvals and Market Access
Data harmonization significantly impacts regulatory approvals and market access in the pharmaceutical industry by streamlining the submission process for new drugs. As the global landscape of healthcare becomes increasingly complex, companies often face varying regulatory requirements across different regions.
This fragmentation can lead to delays in bringing innovative treatments to market. By harmonizing data, companies can create standardized submissions that meet multiple regulatory standards simultaneously, reducing the need for duplicate assessments and extensive documentation.
This streamlined approach not only accelerates the review process but also enhances the quality of data submitted to regulatory bodies. Improved data quality leads to more reliable assessments, facilitating quicker approvals.
Additionally, harmonized data supports better collaboration between regulatory agencies, allowing for shared inspections and evaluations. As a result, patients gain faster access to essential medications.
The importance of data harmonization is further underscored by initiatives from organizations like the Clinical Data Interchange Standards Consortium (CDISC), which aim to establish common standards for data submission.
Ultimately, effective data harmonization is crucial for ensuring that new therapies reach the market efficiently and safely, benefiting both patients and healthcare systems worldwide.
Key Technologies Enabling Data Harmonization in Drug Development
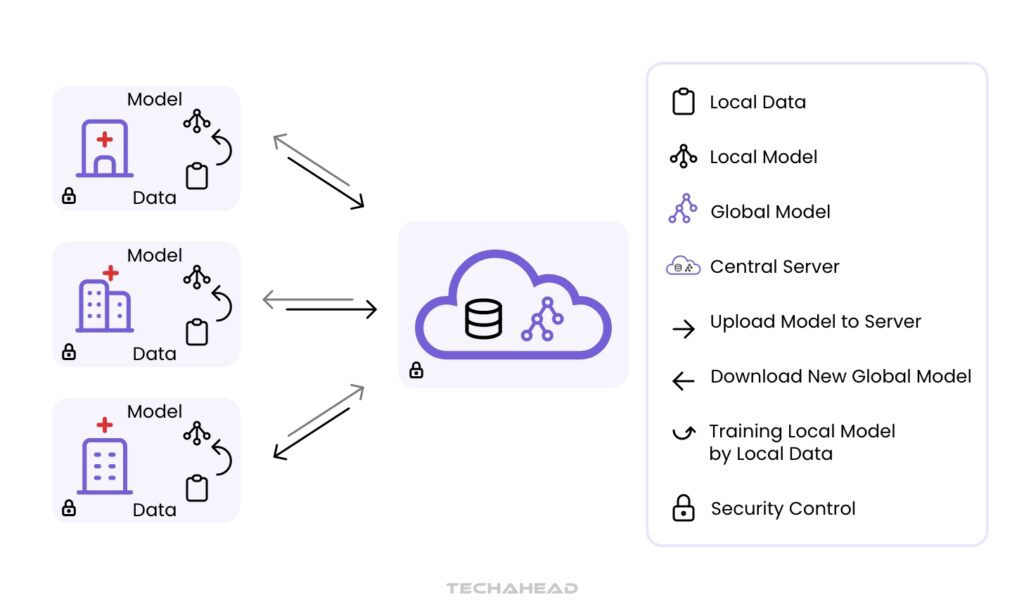
Key technologies enabling data harmonization in drug development include artificial intelligence (AI) and federated learning, which are transforming how researchers handle sensitive patient data. Federated learning allows machine learning models to be trained on decentralized data sources without transferring raw data between institutions.
This approach enhances privacy by enabling local training on patient data at each center, while only sharing model updates with a central server.
As illustrated in the above figure, the central server aggregates these updates to refine a global model, facilitating harmonized analysis across multiple healthcare facilities. This iterative process not only preserves patient confidentiality but also improves the accuracy of predictive models used for optimizing drug allocation strategies and enhancing patient outcomes.
Moreover, the integration of AI techniques with federated learning supports the development of robust analytical frameworks that can analyze large datasets from various sources.
This capability is essential for advancing research in drug development, as it allows for comprehensive insights while maintaining compliance with privacy regulations. By leveraging these technologies, researchers can accelerate innovation and improve decision-making processes in the healthcare sector.
The Role of Standardization in Data Harmonization for Drug Development
Data powers modern drug discovery, driving insights and decisions in early research stages. However, managing vast amounts of diverse data from different phases creates challenges that data harmonization helps solve by standardizing and integrating scattered datasets.
By creating consistent formats and structures across datasets, harmonization improves data quality and supports machine learning applications that ultimately benefit patients. It enables accurate comparisons and strengthens research findings by reducing analysis errors.
Early-stage R&D data comes from many sources, including public databases and lab experiments. These sources often use different formats and structures. Harmonization bridges these gaps, allowing smooth integration that provides better statistical power for analysis and speeds up research.
When researchers align their data to shared frameworks, they can easily share knowledge and work together. This teamwork leads to deeper understanding of complex biological systems. Harmonized data also simplifies workflows, cutting down time spent on data processing and letting researchers use analysis tools more effectively.
However, challenges exist. These include dealing with different data types from various
research techniques, breaking down data silos between departments, handling inconsistent formats, ensuring data quality, and managing large data volumes. Despite these hurdles, harmonization remains vital for advancing drug development through better use of research data.
How to Implement Data Harmonization in Pharmaceutical Research
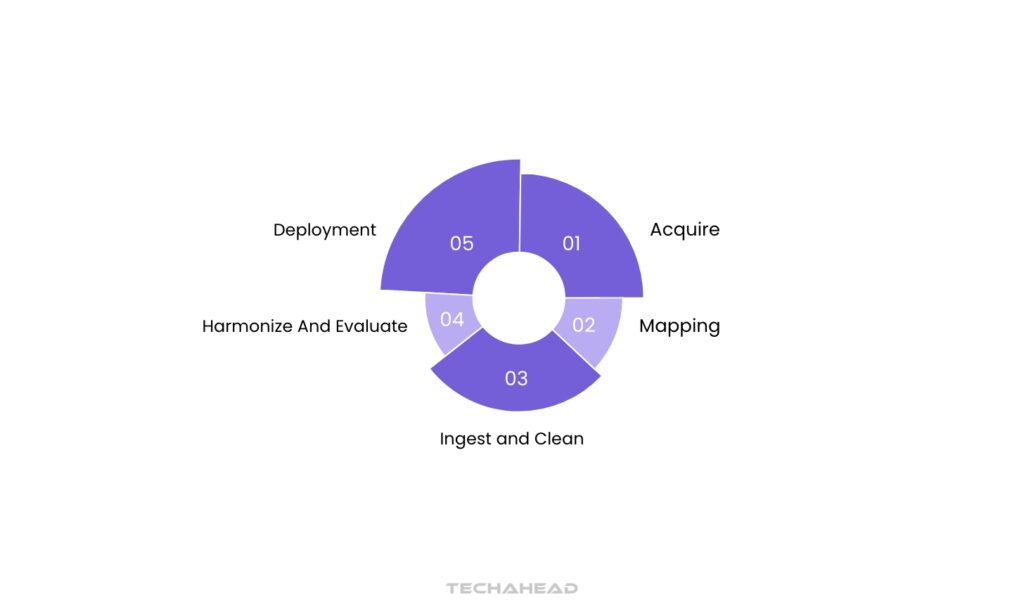
Step 1: Acquire
Begin by identifying and collecting data from various pharmaceutical research sources including clinical trial data, laboratory results, patient records, molecular screening data, and biomarker studies. Create comprehensive datasets that capture both historical and ongoing research information.
Step 2: Mapping
Develop a unified schema that accommodates pharmaceutical-specific data requirements. This schema should include standardized fields for chemical structures, biological targets, dosage information, adverse effects, and experimental conditions. Ensure compliance with industry standards like CDISC and FAIR data principles.
Step 3: Ingest and Clean
Import the raw research data into your system. Validate critical data points like compound identifiers, biological measurements, and experimental parameters. Clean the data by standardizing units of measurement, resolving inconsistent nomenclature, and addressing missing values while maintaining data integrity.
Step 4: Harmonize and Evaluate
Apply the schema to transform raw data into a standardized format. Verify that the harmonized data maintains accuracy in crucial areas like chemical structures, activity values, and experimental conditions. Ensure the harmonization process preserves statistical significance and biological relevance.
Step 5: Deployment
Make the harmonized data available across research teams through a centralized platform. Enable secure access for different stakeholders including chemists, biologists, and clinical researchers. Implement version control and audit trails to track data modifications while maintaining regulatory compliance.
The Importance of Cross-Industry Collaboration for Data Harmonization
Cross-industry collaboration is essential for effective data harmonization, particularly in the realm of healthcare and research. As various sectors work to streamline data sharing workflows, it becomes clear that collaboration between data repositories and journals can significantly enhance the efficiency of these processes.
Currently, differing practices across industries often hinder seamless data exchange, leading to delays and misunderstandings.
By fostering communication among stakeholders, organizations can identify challenges and develop standardized guidelines that facilitate better data sharing. This collaboration can result in a unified approach to data management, ultimately benefiting researchers and enhancing the quality of scientific outputs.
The goals of such initiatives include creating a comprehensive list of challenges faced by different groups and producing guidelines or white papers that outline best practices for data sharing.
As these partnerships evolve, they pave the way for modernized procedures that not only ease data sharing but also promote innovation in research communications. Ultimately, cross-industry collaboration is vital for advancing knowledge creation and ensuring that valuable data is utilized effectively across various fields.
The Economic Impact of Data Harmonization in Pharmaceutical R&D
Data harmonization significantly reduces costs and improves efficiency in pharmaceutical research and development. By standardizing data formats and eliminating redundant data collection efforts, organizations can save substantial resources previously spent on managing inconsistent datasets.
This streamlined approach reduces the time researchers spend on data preparation and analysis, allowing faster progression through development stages. Harmonized data also minimizes expensive errors and research delays caused by data inconsistencies, while enabling better collaboration between research teams.
Furthermore, standardized data supports more efficient regulatory submissions, potentially accelerating time-to-market for new drugs. The improved data quality and accessibility also enhance decision-making processes, leading to better resource allocation and increased return on investment in research programs.
Conclusion
In conclusion, data harmonization is crucial for enhancing machine learning models in drug development. By ensuring that diverse datasets are standardized and integrated, researchers can train models on high-quality data, leading to more accurate predictions and improved patient outcomes. This process not only accelerates the drug discovery timeline but also fosters collaboration among research teams, enabling them to leverage comprehensive insights. As the pharmaceutical landscape continues to evolve, prioritizing data harmonization will be essential for maximizing the potential of machine learning and driving innovation in healthcare.
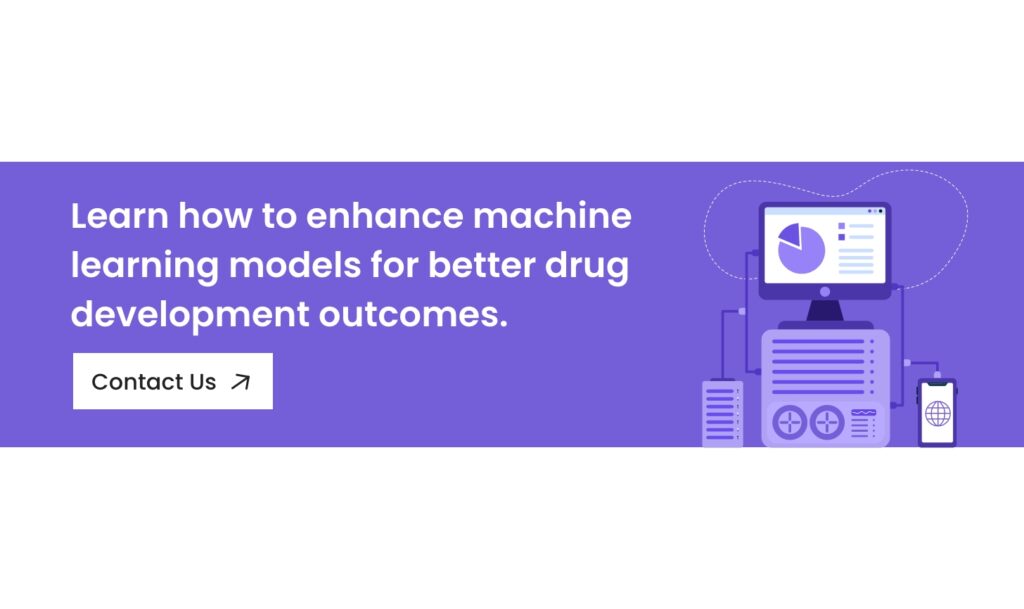
FAQ
To achieve data harmonization, organizations should implement consistent data collection methods, use shared data structures, and invest in powerful analytical tools that enable seamless data integration and analysis.
Federated learning enables training of machine learning models on data stored across various devices or locations. This approach protects user privacy and fosters collaboration without needing to centralize sensitive information.
Metadata acts like a label for data, providing essential information about its format and what it contains. This context simplifies combining and analyzing data from various sources, making it more usable and understandable.