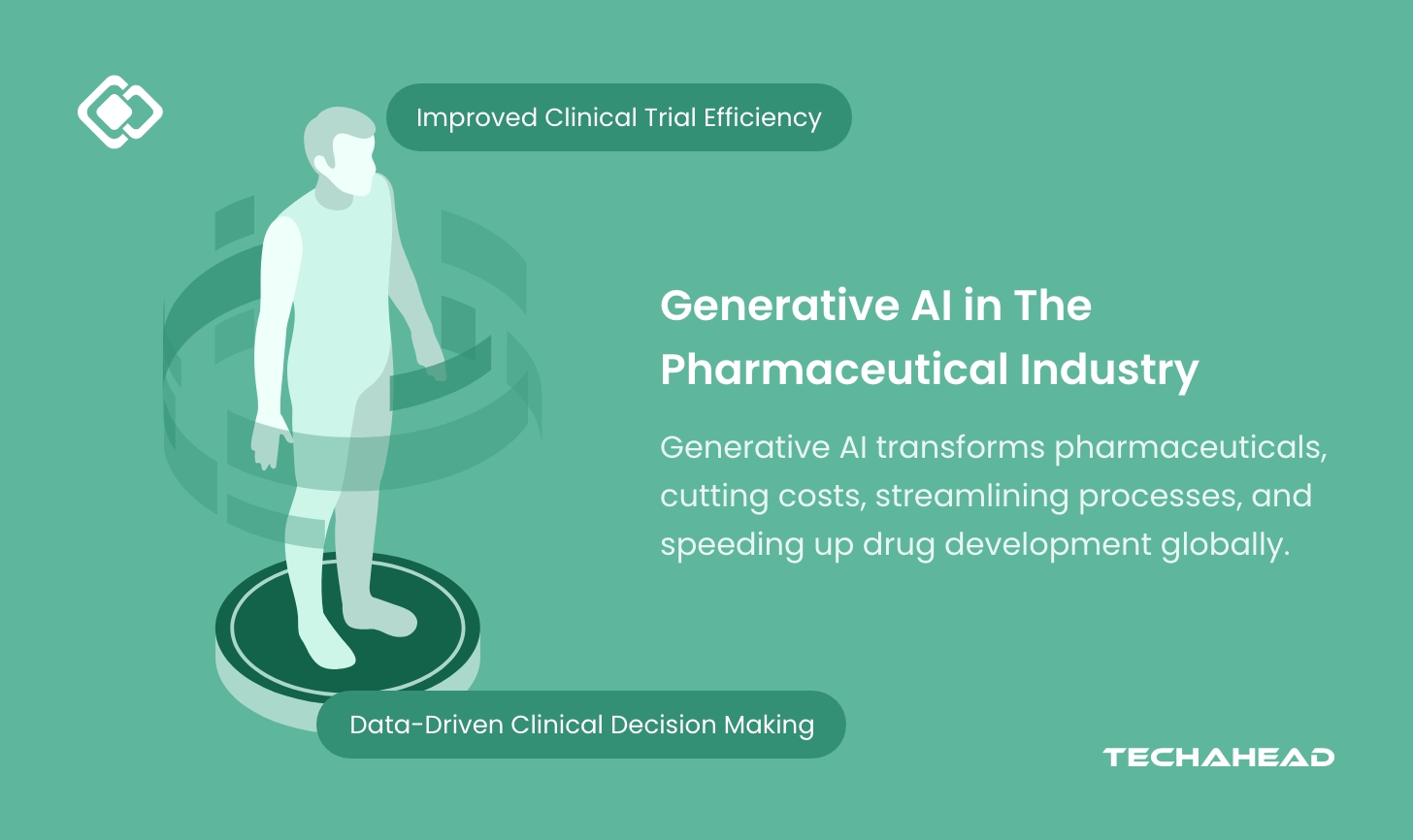
The pharmaceutical industry stands on the brink of a transformative revolution, with Generative AI poised to unlock an astounding $60 billion to $110 billion in annual economic value. As companies grapple with the staggering costs of drug development—averaging $2.3 billion and taking 10 to 15 years—many are turning to innovative technologies like Generative AI to streamline processes and enhance efficiency.
With only 10% of candidate drugs successfully reaching the market, the urgency for change has never been greater.
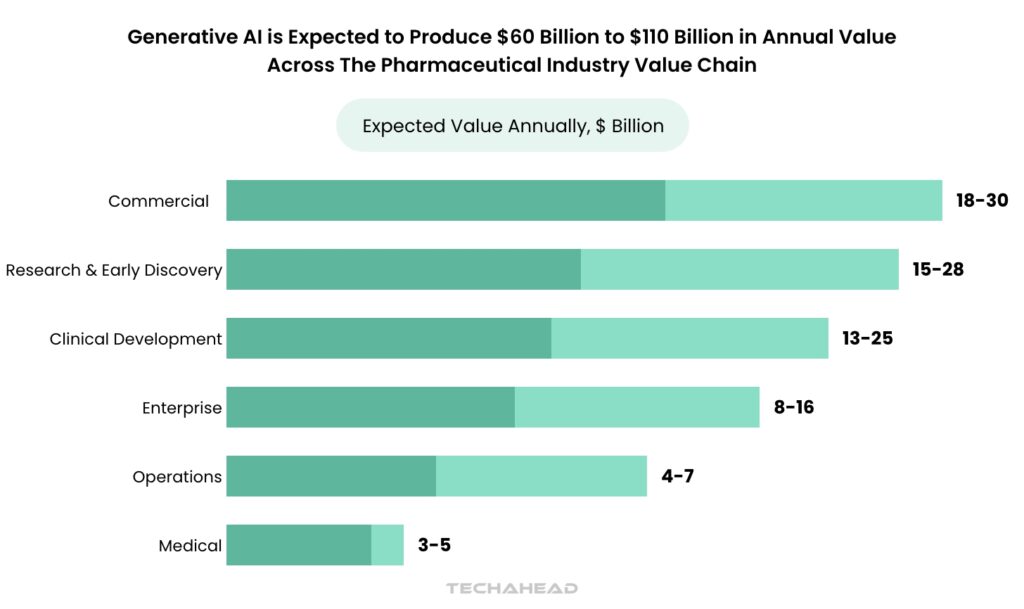
Generative AI gives a game-changing solution, accelerating drug discovery, optimizing clinical trials, and automating workflows from improvement to advertising and marketing. Imagine a global where researchers can unexpectedly pick out promising compounds, regulatory approvals are expedited, and personalized remedies are tailored to personal patient desires—all made possible through harnessing the energy of AI.
In this blog, we are able to discover how Generative AI is reshaping the pharmaceutical landscape, addressing each of its extraordinary benefits and the demanding situations that include integrating this modern-day era into existing workflows.
Join us as we delve into this interesting frontier and find out how the future of pharmaceuticals is being redefined.
Understanding the Realities of Generative AI in Pharma: Dispelling Common Hype
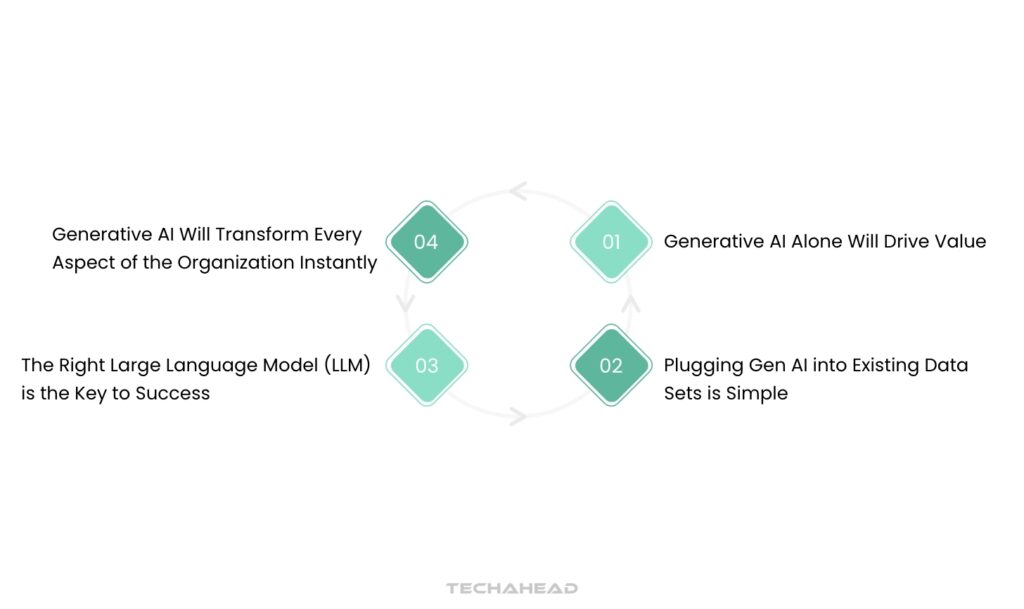
Before pharmaceutical companies can effectively leverage the opportunities presented by Generative AI (Gen AI), it is crucial to differentiate between the realities of this technology and the surrounding hype. Here, we clarify four prevalent misconceptions that business leaders may hold about Gen AI.
Hype 1: Generative AI Alone Will Drive Value
Reality: While Gen AI represents a significant advancement, it is part of a broader landscape of artificial intelligence. Traditional analytical models will continue to provide value, but Gen AI applications will enhance their capabilities, leading to greater overall impact.
Hype 2: Plugging Gen AI into Existing Data Sets is Simple
Reality: Successful implementation of Gen AI requires a well-structured data architecture. Companies must develop an intelligence layer capable of understanding complex data types, including molecular structures and patient information. This involves collaboration among data scientists, business strategists, and compliance experts to establish a robust data infrastructure.
Hype 3: The Right Large Language Model (LLM) is the Key to Success
Reality: According to research, LLMs account for only about 15% of the effort in a typical Gen AI project. The majority of work involves adapting these models to fit a company’s unique internal knowledge and regulatory requirements. Effective integration into existing workflows is essential for maximizing the technology’s impact.
Hype 4: Generative AI Will Transform Every Aspect of the Organization Instantly
Reality: Digital transformation requires careful prioritization. Leaders should focus on specific use cases that align with overall business goals and create a strategic roadmap for implementation. A balanced approach—starting with low-disruption projects while planning for more transformative initiatives—will optimize impact and ensure successful adoption.
Use Cases of Generative AI in Pharmaceutical Industry
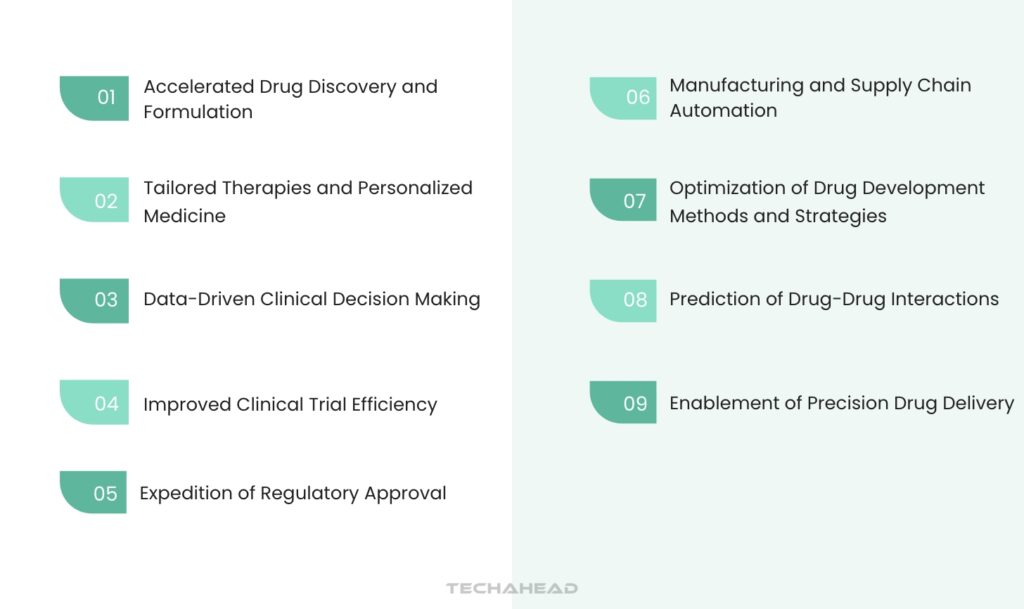
Generative AI is poised to revolutionize the pharmaceutical industry, impacting everything from drug discovery to regulatory approval. Here are some key use cases:
Accelerated Drug Discovery and Formulation
Generative AI significantly accelerates the traditional drug discovery process. By leveraging machine learning algorithms and advanced data analytics, AI can sift through vast amounts of biological, chemical, and clinical data to identify promising drug candidates more efficiently. This includes:
- Virtual Screening: Using computational simulations, AI can screen massive chemical databases to identify potential drug candidates, drastically reducing the time and cost of initial screening.
- Molecular Modeling: AI employs computational techniques to simulate and analyze molecular structures, aiding in the design and optimization of potential drug compounds for improved efficacy and reduced side effects.
- Predictive Analytics: Statistical algorithms analyze large datasets to predict outcomes, facilitating the identification of promising drug candidates and potential drug-target interactions.
Tailored Therapies and Personalized Medicine
Generative AI is revolutionizing the pharmaceutical industry by enabling the development of personalized medicine through the analysis of diverse patient datasets, including genetic information, medical histories, and lifestyle factors. This capability allows for:
- Targeted Therapy Design: AI models analyze individual patient data to identify specific molecular targets and develop customized treatment regimens optimized for their unique biological characteristics.
- Biomarker Identification and Treatment Response Prediction: AI identifies relevant biomarkers and predicts individual treatment responses, enabling the creation of tailored treatment plans that maximize therapeutic efficacy and minimize adverse effects. This moves away from the traditional “one-size-fits-all” approach to healthcare.
Optimization of Drug Development Methods and Strategies
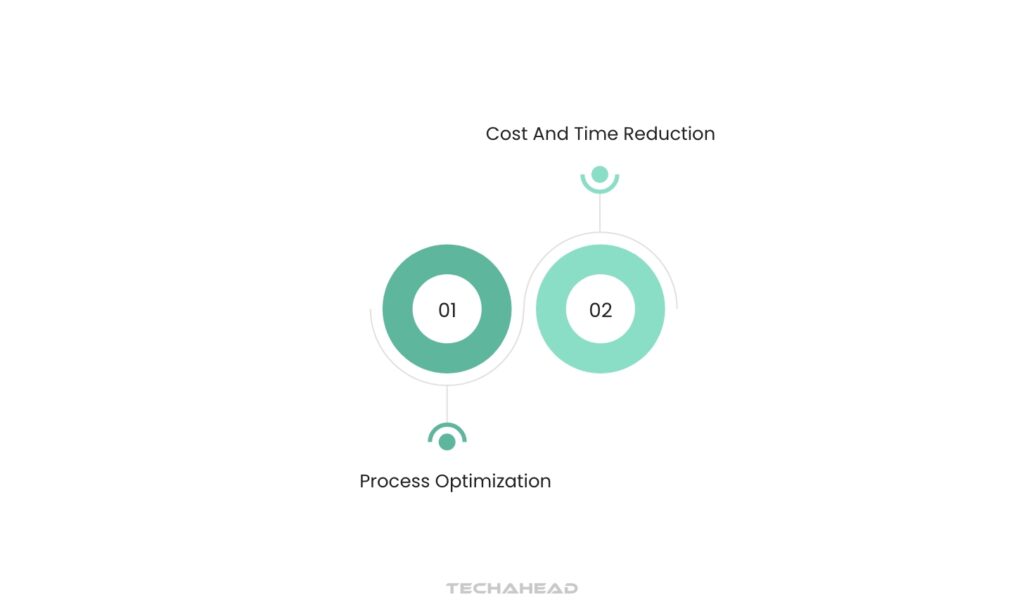
Generative AI is revolutionizing drug development by streamlining processes and enhancing efficiency. This technology enables faster drug discovery, accelerates clinical trials, and optimizes regulatory approvals, ultimately leading to quicker market entry for new therapies.
- Process Optimization: AI models can analyze large datasets and discover methods to optimize drug development methodologies, streamlining workflows and reducing bottlenecks.
- Cost and Time Reduction: This improved approach significantly reduces research costs and shortens the time-to-market for new medications, making treatments more accessible to patients.
Data-Driven Clinical Decision Making
Generative AI is revolutionizing healthcare by providing healthcare providers with invaluable data insights. This technology enables the analysis of vast amounts of patient data, leading to more accurate diagnoses and personalized treatment plans, ultimately enhancing patient care and outcomes.
- Comprehensive Data Analysis: AI analyzes diverse medical parameters, including patient demographics, treatment responses, and disease progression, to generate valuable insights.
- Evidence-Based Medicine: This data-driven approach supports informed clinical decision-making and promotes evidence-based medicine, leading to better patient care.
Manufacturing and Supply Chain Automation
Generative AI is revolutionizing pharmaceutical manufacturing and supply chain operations by enhancing efficiency, reducing costs, and streamlining processes. This technology enables real-time data analysis, predictive maintenance, and optimized inventory management, ultimately leading to faster drug production and improved delivery timelines.
- Automation of Key Processes: AI automates various tasks, including production scheduling, inventory management, and distribution logistics, optimizing the entire supply chain.
- Cost Reduction and Efficiency: This automation enhances efficiency, reduces operational expenses, minimizes waste, and ensures the timely delivery of medications, improving access for patients.
Improved Clinical Trial Efficiency
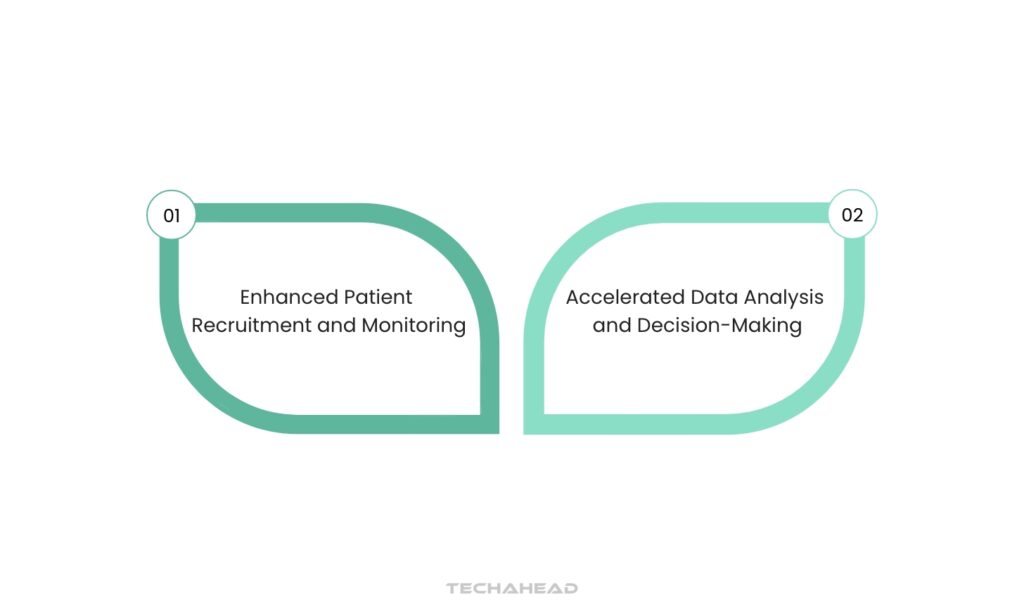
Generative AI is revolutionizing the pharmaceutical industry by optimizing various aspects of clinical trials, including patient recruitment, data analysis, and trial design. This technology accelerates processes, enhances efficiency, and improves outcomes, ultimately transforming how drugs are developed and brought to market.
- Enhanced Patient Recruitment and Monitoring: AI assists in patient recruitment, selection, and monitoring, improving trial enrollment and retention rates.
- Accelerated Data Analysis and Decision-Making: AI aids in data analysis and interpretation, facilitating faster decision-making and reducing overall trial duration, bringing new treatments to market more quickly.
Prediction of Drug-Drug Interactions
Generative AI examines extensive data on drug interactions and side effects to identify potential risks and predict how different medications might interact, helping prevent adverse reactions and improve patient safety.
- Identify Potential Risks: AI models can accurately predict potential drug-drug interactions before they occur, helping to prevent adverse events.
- Inform Treatment Decisions: This predictive capability enables healthcare providers to make informed treatment decisions and avoid potentially harmful drug combinations, improving patient safety.
Enablement of Precision Drug Delivery
Generative AI enables tailored drug delivery systems by analyzing individual patient data, such as medical history and health status. This personalization maximizes treatment effectiveness while minimizing potential side effects.
- Customized Drug Delivery Systems: AI can customize drug delivery systems based on individual patient needs, considering their health and medical history.
- Maximized Efficacy and Minimized Side Effects: This personalized approach minimizes the risk of side effects and maximizes treatment efficacy, improving patient outcomes.
Expedition of Regulatory Approval
Generative AI simplifies regulatory processes by automating tasks like generating comprehensive documentation and ensuring compliance with evolving guidelines. This automation reduces administrative burdens for both pharmaceutical companies and regulatory agencies, accelerating the review and approval of new therapies.
- Automating Regulatory Submissions: AI generates comprehensive regulatory submissions, ensuring compliance and speeding up the review and approval process.
- Reduced Administrative Burden: This automation reduces administrative burdens for both pharmaceutical companies and regulatory agencies, enabling faster access to innovative treatments for patients.
Key Considerations for Each Aspect of Scaling Gen AI
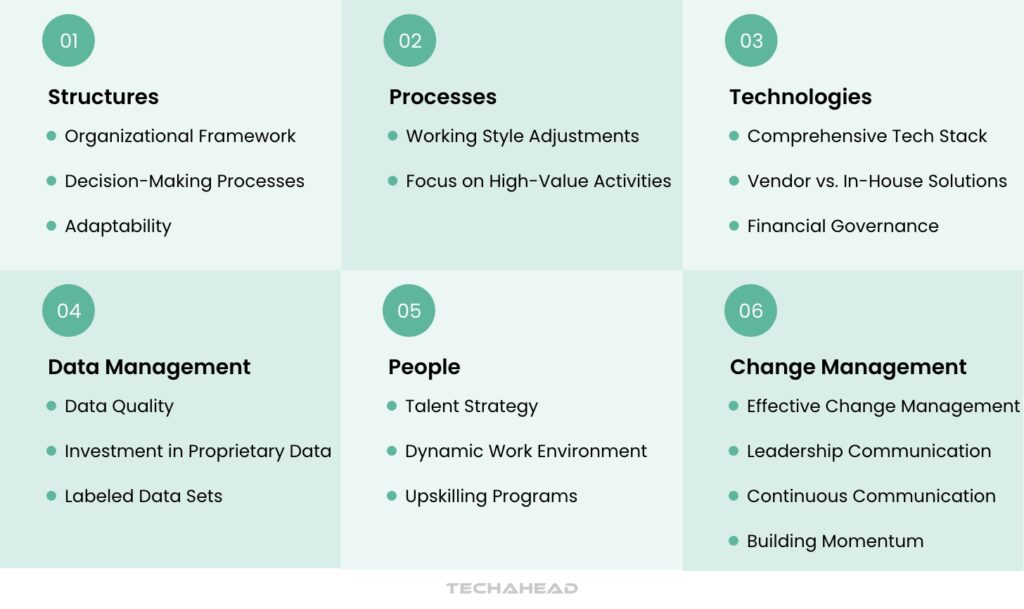
To effectively integrate Generative AI (Gen AI) into the pharmaceutical sector, organizations must establish a robust framework that encompasses structures, processes, technologies, data management, people, and change management. Below are the key components that should be considered:
Structures
- Organizational Framework: Define an organizational structure that aligns with strategic goals, such as adopting a product-centric approach or establishing a centralized Center of Excellence (CoE). This structure should facilitate collaboration and innovation.
- Decision-Making Processes: Establish clear governance and decision-making protocols for critical issues, including roles, responsibilities, and workforce size. This ensures accountability and efficient operations.
- Adaptability: The organization must remain flexible to allow structures to evolve in response to changing needs and advancements in Gen AI technology.
Processes
- Working Style Adjustments: Implement both incremental changes and fundamental shifts in working styles. Professionals across various roles—strategists, researchers, and creatives—must adapt their approaches to leverage Gen AI’s capabilities fully.
- Focus on High-Value Activities: Effective implementation of Gen AI can free up employees for more strategic tasks, reshaping roles throughout the organization to enhance productivity.
Technologies
- Comprehensive Tech Stack: While large language models (LLMs) like ChatGPT are foundational, they represent only a fraction of a complete Gen AI solution. Organizations need to design a comprehensive tech stack tailored to their specific needs regarding information security, performance, and latency.
- Vendor vs. In-House Solutions: Striking a balance between developing in-house solutions and utilizing vendor-provided technologies is crucial for optimizing resources.
- Financial Governance: Robust financial governance and FinOps practices are essential for managing the costs associated with Gen AI implementation effectively.
Data Management
- Data Quality: The effectiveness of Gen AI relies on high-quality data. Organizations must continuously enrich their data and ensure proper sharing across internal functions.
- Investment in Proprietary Data: Strategic differentiation may require investments in proprietary datasets to enhance the capabilities of Gen AI applications.
- Labeled Data Sets: Building and managing labeled datasets is essential for measuring and tracking the performance of Gen AI applications while addressing existing data quality issues.
People
- Talent Strategy: The rapid pace of change necessitates a proactive talent strategy to address skill gaps. This may involve recruiting new talent, upskilling existing employees, or reallocating resources based on evolving priorities.
- Dynamic Work Environment: Attracting and retaining AI talent requires a modern tech stack and an agile working model that fosters experimentation and continuous skill development.
- Upskilling Programs: Implementing comprehensive upskilling programs led by knowledgeable leaders is vital for preparing the workforce for the demands posed by Gen AI.
Change Management
- Effective Change Management: Successful adoption of Gen AI at scale hinges on effective change management strategies that promote shifts in mindsets and behaviors across the organization.
- Leadership Communication: Leaders must actively communicate the transformation narrative, build awareness and excitement about Gen AI initiatives, and address any concerns from stakeholders.
- Continuous Communication: Maintaining open lines of communication is crucial for sustained adoption of Gen AI technologies. Training employees on the strengths, limitations, risks, and proper prompt structuring is essential before rollout.
- Building Momentum: Creating teams of early adopters can help shape the deployment of Gen AI use cases, demonstrating their value and building momentum for broader acceptance within the organization.
Generative AI in Pharma Manufacturing and Regulatory Compliance
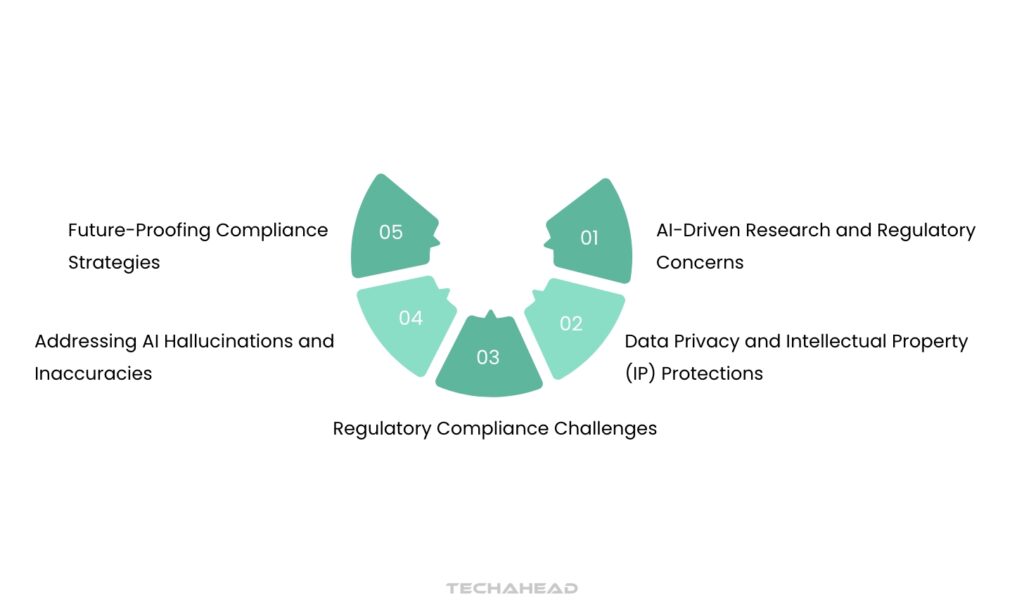
Generative AI has the potential to revolutionize pharma manufacturing, but it also introduces significant risks, particularly related to regulatory compliance and intellectual property (IP) concerns.
As generative AI systems become increasingly integrated into the pharmaceutical industry, particularly in the U.S., it’s critical to understand the implications of these risks and how they can be managed within a highly regulated environment.
Here are some key considerations for pharma companies deploying generative AI in manufacturing, research, and regulatory compliance:
Regulatory Compliance Challenges:
- The U.S. FDA, alongside other national regulatory bodies, enforces strict standards on the development, manufacturing, and marketing of pharmaceutical products.
- Generative AI models must adhere to these established regulations, including Good Manufacturing Practices (GMP), which emphasize product safety, quality, and integrity.
- With generative AI influencing the design and testing of pharmaceutical products, ensuring AI-driven processes comply with FDA guidelines is essential to avoid costly setbacks or delays.
- Emerging AI regulations, including the AI Risk Management Framework by the FDA and state-specific laws, are still evolving. As these regulations change, it is crucial to stay ahead of compliance requirements and ensure AI models are aligned with these evolving rules.
Data Privacy and Intellectual Property (IP) Protections:
- In the pharmaceutical industry, where patient privacy is paramount, generative AI systems must be designed to handle sensitive medical data in compliance with regulations like HIPAA (Health Insurance Portability and Accountability Act).
- Many AI models rely on data collected from the internet, posing the risk of inadvertent IP infringement, particularly when dealing with proprietary pharmaceutical formulations, clinical trial data, or trade secrets.
- Pharma companies must implement robust IP protections, such as using AI systems trained exclusively on their own proprietary data, ensuring compliance with data privacy laws while mitigating the risk of accidental data leakage or plagiarism.
Addressing AI Hallucinations and Inaccuracies:
- One of the key concerns with generative AI is the risk of “hallucinations” or false outputs, particularly in the context of pharmaceutical research and manufacturing.
- While AI can suggest innovative treatment avenues or manufacturing optimizations, hallucinations or inaccuracies can result in costly errors or non-compliance with established safety protocols.
- To mitigate these risks, pharma companies should incorporate human oversight into all AI-driven decision-making processes, ensuring that AI-generated outputs are reviewed by qualified experts before being implemented in the production process.
AI-Driven Research and Regulatory Concerns:
- In research and development, generative AI can assist in discovering new drug formulations or accelerating clinical trial processes. However, AI’s ability to suggest novel compounds or treatment methods must be evaluated against stringent regulatory standards before being pursued in clinical trials.
- For example, new treatments must undergo rigorous safety testing and approval by the FDA before they can be marketed. AI can help streamline this process, but the final decision on the safety and efficacy of a new treatment always rests with human experts and regulatory bodies.
Future-Proofing Compliance Strategies:
- As generative AI continues to evolve, so too will the regulatory landscape. To maintain compliance, pharma companies should proactively engage in cross-disciplinary risk management, integrating AI governance frameworks that include legal, regulatory, and ethical considerations.
- The adoption of AI must be accompanied by careful tracking and documentation of AI models’ decision-making processes, ensuring traceability and accountability for every step, from research to manufacturing.
Challenges and Ethical Considerations in Generative AI for Pharma
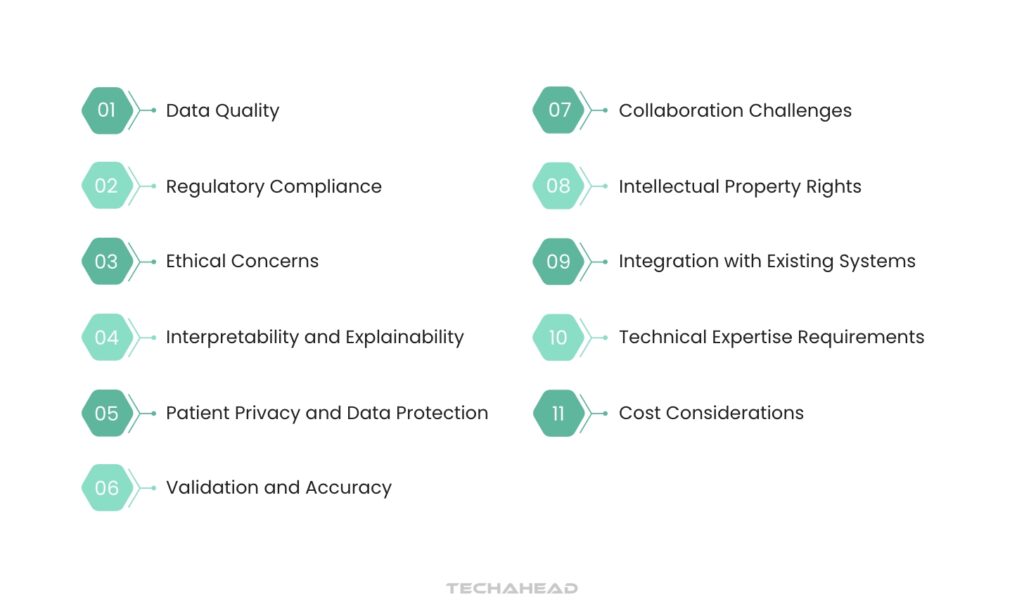
The integration of Generative AI into the pharmaceutical industry presents significant challenges and ethical considerations that must be addressed to harness its full potential effectively. Here are key points to consider:
Data Quality
The effectiveness of Generative AI models heavily relies on the quality of data used for training. Poor-quality or biased data can lead to inaccurate predictions and ineffective drug designs, potentially compromising patient safety and treatment efficacy.
Regulatory Compliance
Pharmaceuticals operate under strict regulatory frameworks. The introduction of Generative AI technologies must comply with regulations set by bodies like the FDA, which can be a lengthy and costly process. Ensuring that AI-generated drugs meet safety and efficacy standards is paramount.
Ethical Concerns
The potential misuse of Generative AI raises ethical questions, particularly regarding the creation of drugs without adequate testing. There are concerns about developing treatments that may be effective only for specific demographics, leading to healthcare disparities and inequality in access.
Interpretability and Explainability
Many Generative AI models function as “black boxes,” making it difficult to understand their decision-making processes. In an industry where transparency is crucial for regulatory approvals and ethical considerations, ensuring model interpretability is a significant challenge.
Patient Privacy and Data Protection
The use of patient data in training AI models raises concerns about privacy and data security. Safeguarding sensitive health information while utilizing it for model training is essential to maintain patient trust.
Validation and Accuracy
Before deploying Generative AI models for critical pharmaceutical tasks, rigorous validation is necessary to ensure their accuracy and reliability. Establishing robust validation methodologies tailored to the pharmaceutical domain can be complex and time-consuming.
Collaboration Challenges
Successful implementation of Generative AI requires collaboration between AI experts, pharmaceutical researchers, and regulatory specialists. Bridging the gap between these diverse fields can be challenging but is essential for effective integration.
Intellectual Property Rights
The application of Generative AI raises questions regarding intellectual property ownership of drugs developed through this technology. Clarifying these rights is necessary to encourage innovation while protecting creators’ interests.
Integration with Existing Systems
Incorporating Generative AI into established pharmaceutical systems can be difficult due to their complexity. Companies may need to invest in new infrastructure to support AI technologies effectively.
Technical Expertise Requirements
Developing and implementing Generative AI models necessitates advanced technical skills. Pharmaceutical companies may need to recruit personnel with expertise in machine learning, deep learning, and data science to navigate this landscape successfully.
Cost Considerations
The initial investment required for developing and implementing Generative AI can be substantial. Companies must carefully evaluate the costs against potential benefits before committing resources to this technology.
Conclusion
In conclusion, the integration of Generative AI into the pharmaceutical industry holds immense potential to transform drug discovery and development, significantly reducing the time and cost associated with bringing new therapies to market. However, realizing this potential requires overcoming substantial challenges, including the need for diverse datasets and robust validation processes.
As the historical analogy of electricity illustrates, immediate productivity gains may not be evident; instead, a thoughtful approach is necessary to fully leverage Gen AI’s capabilities. Pharmaceutical companies must develop comprehensive strategies that encompass structures, processes, technologies, data management, and change management to ensure successful implementation.
By fostering a culture of innovation and adaptability, and by investing in talent development and collaboration across disciplines, organizations can navigate the complexities of Gen AI adoption. While the journey may be gradual, the long-term benefits—improved patient outcomes and enhanced efficiency—make it a worthwhile endeavor for the future of healthcare.
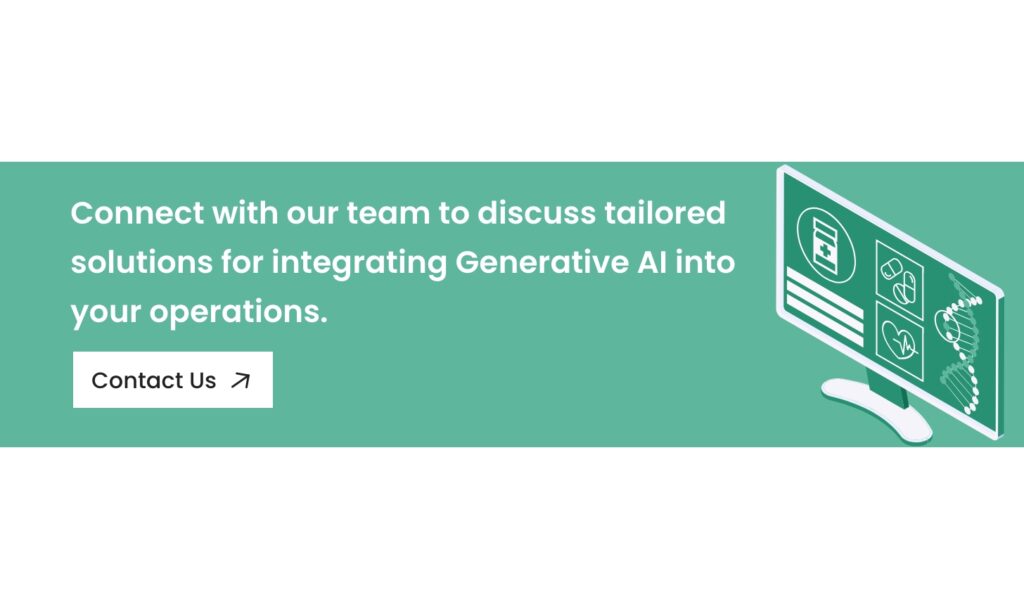
FAQ
Future trends point towards more personalized treatments tailored to individual patients, enhanced predictive analytics for better forecasting of patient outcomes, and wider integration of these technologies throughout the entire drug development lifecycle, from discovery to delivery.
Set clear goals everyone can understand, keep team meetings regular and honest, and check often to see if the work matches what you planned. Make sure people talk to each other and track how well things are going.
To ensure responsible AI implementation, organizations need clear data usage guidelines, robust model validation procedures, and complete transparency throughout the entire development process. This builds trust and ensures ethical AI practices.